CDSS for Sleep Staging (2020.09)
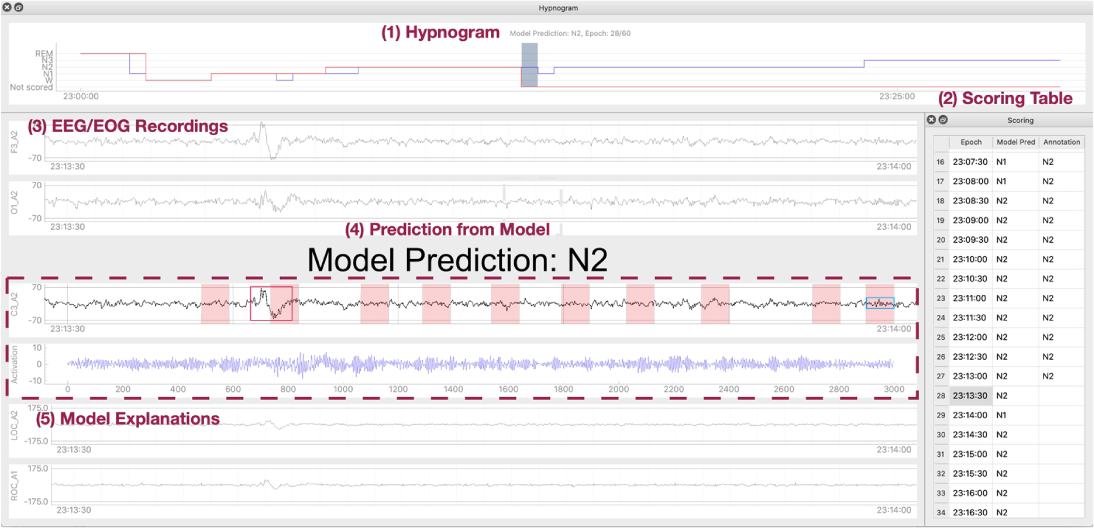
We developed a clinical decision support system (CDSS) to assist polysomnographic technicians by providing information on important EEG features in the given EEG segments. In this work, we extracted information from deep learning models and constructed visualization strategies in a user-centered approach.
Problem Definition
Recent breakthroughs in deep learning algorithms have brought unprecedented performances in automating clinical diagnosis. However, full automation of the diagnosis is still not available as we need to validate the stability of those algorithms in several aspects. Under these circumstances, artificial intelligence (AI) models are primarily designed as clinical decision support systems (CDSSs) and reviews of algorithmic predictions by human experts remain mandatory.
Lack of interpretability in deep learning models has been one of the biggest challenges in adopting these models in clinical domains. Therefore, information systems need to provide sets of desired information defined from the domains adequately. This project aims to construct a CDSS for sleep staging that helps technicians efficiently review AI predictions by providing information regarding how AI predicted the sleep stages and whether this correctly corresponds to domain knowledge in sleep medicine.
Approach
Our development process included three phases: (1) interviews with polysomnographic technicians to identify why users might desire explanations from the CDSS when adopting AI-based sleep scoring systems; (2) user observations of how polysomnographic technicians score sleep stages from EEG recordings to determine the information that could help them; and (3) an iterative design process to construct a user-friendly CDSS interface that addresses how explanations should be formulated in the system.
User interview
We conducted user interviews with polysomnographic technicians and identified user needs on which information is needed for reviewing sleep staging results from algorithmic systems. We explicitly investigated in which context explanations from AI were desired in practice. Furthermore, we asked how they perceived conventional automatic sleep staging systems.
As stated in the subsection above, technicians requested that AI programs should provide clinically sound explanations for predictions since reviewing the correctness of AI predictions without this information is no different from the manual annotation of sleep stages from scratch. To summarize the trend of the interview answers, the technicians wanted explanations to validate the correctness of the AI predictions based on their clinical knowledge on sleep staging.
User observation
A user observation study was performed to understand the sleep staging conventions of clinical practitioners. From the observed sleep staging conventions, we aimed to construct a list of EEG characteristics to which technicians refer. During this study, hour-long weekly meetings were held over a month in which a participating technician scored EEG epochs in a think-aloud protocol.
By observing a technician for a month, we obtained an understanding of how technicians interpret EEG signals during sleep staging. Using the clinical context proposed in the manual [1], we categorized EEG patterns based on how the technician processed the information in EEG recordings. Based on how they processed each EEG feature, we made a list of explanation types that can be provided in the CDSS.
Iterative design
We conducted an iterative design process with a technician to identify how explanations should be presented to the CDSS users. For two months, we held weekly two-hour meetings.
Visualization strategies for each clinical feature were devised to provide information in an easily adopted form for sleep staging. Initially, plots of activation vectors without any processing were provided to the participating technician. In this case, the technician failed to use any of the information in the activation values. They emphasized that information should be compatible with the technician’s scoring procedure. From this standpoint, we constructed different visualization strategies for each explanation type since conventions observed during the user observation study constituted the representative logical procedures for processing information in EEG recordings.
Results
After development, polysomnographic technicians performed quantitative and qualitative evaluations of the system. During the iterations, we aimed to investigate whether the information contained in the above components could provide the desired information that was obtained from the user observation study. In these sessions, the technician inspected the features obtained by the neural network components and expressed an opinion on whether they could provide sufficient explanation for the task. Information from the components was refined based on the feedback. Afterward, we chose the exact component for generating explanations from neural network components. However, because the information in neural networks is numerical, adequate visualization is required to enhance the user-friendliness of the explanations. Therefore, we iteratively collected feedback on the representation format of the explanations during the later sessions. The technician tested the prototype versions of the proposed tool and provided feedback in terms of their intuitiveness and helpfulness. Consequently, visualization strategies were constructed for the explanations and overall interfaces.
Quantitative evaluation
When evaluating the improvements in the sleep staging performance of all participants, we did not observe significant improvements where the P-value was approximately .17. However, we still believe that our quantitative evaluations contain meaningful results. First, when assessing the improvements for novice participants, we observed that the Macro-F1 scores improved by 6.7% with a P-value of .02. Considering that novice technicians may rely more on supportive information than expert technicians, this result implies that our tool could be effectively used to augment the sleep scoring capacities of novice technicians with acceptable sleep-relevant explanations. Second, when assessing the improvements in a single-epoch sleep scoring setting, which is similar to a stress-test configuration, we observed significant improvements in the Macro-F1 scores and inter-rater reliability. Notable results in this stress test setting could indicate that our explanations to an extent helped technicians interpret the signal characteristics of each EEG epoch.
Qualitative evaluation
The results from the qualitative evaluation implied that the CDSS supports sleep staging by reducing the workload required for pattern recognition and by providing quantitative visual references. These findings show that the developed system successfully and appropriately complemented the technicians’ assessments by suggesting desired information. Furthermore, participants constructed strategies to successfully collaborate with the AI [43]. To be specific, users evaluated the convincing and unconvincing contributions of the AI, thus efficiently allocating their attention during the adoption.
Publications
For further details on the project, please refer to the following papers published from the project.
- Jeonghwan Hwang*, Taeheon Lee\*, Honggu Lee, and Seonjeong Byun. “Clinical Decision Support System for Sleep Staging Tasks with Explanations from Artificial Intelligence: User-Centered Design and Evaluation.” JMIR Preprints. 09/03/2021:28659
(* indicates co-first author)